Enhancing Security and Asset Management with AI/ML in Cato Networks’ SASE Product
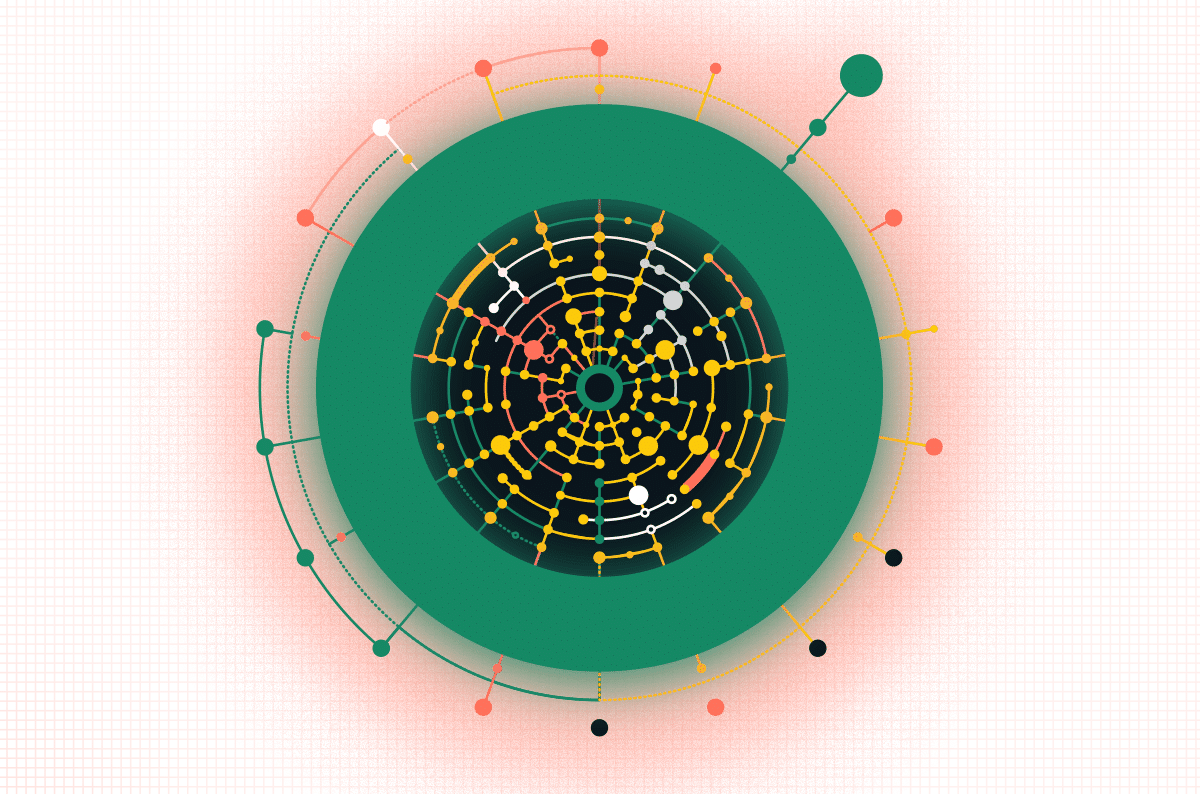
Table of Contents
- 1. What is Artificial Intelligence (AI), Machine Learning (ML), and Deep Learning (DL)?
- 2. Overcoming Challenges in Implementing AI/ML for Real-time Network Security Monitoring
- 3. How Cato Uses Deep Learning to Enhance Threat Detection and Prevention
- 4. How Cato Uses AI and ML in Asset Visibility and Risk Assessment
- 5. Cato Will Continue to Expand its ML/AI Usage
Listen to post:
Getting your Trinity Audio player ready...
|
We just introduced what we believe is a unique application of real-time, deep learning (DL) algorithms to network prevention. The announcement is hardly our foray into artificial intelligence (AI) and machine learning (ML). The technologies have long played a pivotal role in augmenting Cato’s SASE security and networking capabilities, enabling advanced threat prevention and efficient asset management. Let’s take a closer look.
What is Artificial Intelligence (AI), Machine Learning (ML), and Deep Learning (DL)?
Before diving into the details of Cato’s approach to AI, ML, and DL, let’s provide some context around the technologies. AI is the overarching concept of creating machines that can perform tasks typically requiring human intelligence, such as learning, reasoning, problem-solving, understanding natural language, and perception. One example of AI applications is in healthcare, where AI-powered systems can assist doctors in diagnosing diseases or recommending personalized treatment plans.
ML is a subset of AI that focuses on developing algorithms to learn from and make predictions based on data. These algorithms identify patterns and relationships within datasets, allowing a system to make data-driven decisions without explicit programming. An example of an ML application is in finance, where algorithms are used for credit scoring, fraud detection, and algorithmic trading to optimize investment strategy and risk management.
Deep Learning (DL) is a subset of ML, employing artificial neural networks to process data and mimic the human brain’s decision-making capabilities. These networks consist of multiple interconnected layers capable of extracting higher-level features and patterns from vast amounts of data. A popular use of DL is seen in self-driving vehicles, where complex image recognition algorithms allow the vehicle to detect and respond appropriately to traffic signs, pedestrians, and other obstacles to ensure safe driving.
Overcoming Challenges in Implementing AI/ML for Real-time Network Security Monitoring
Implementing DL and ML for Cato customers presents several challenges. Cato handles and monitors terabytes of customer network traffic daily. Processing that much data requires a tremendous amount of compute capacity. Falsely flagging network activity as an attack could materially impact our customer’s operations so our algorithms must be incredibly accurate. Additionally, we can’t interfere with our user’s experience, leaving just milliseconds to perform real-time inference.
Cato tackles these challenges by running our DL and ML algorithms on Cato’s cloud infrastructure. Being able to run in the cloud enables us to use the cloud’s ubiquitous compute and storage capacity. In addition, we’ve taken advantage of cloud infrastructure advancements, such as AWS SageMaker. SageMaker is a cloud-based platform that provides a comprehensive set of tools and services for building, training, and deploying machine learning models at scale. Finally, Cato’s data lake provides a rich data set, converging networking metadata with security information, to better train our algorithms.
With these technologies, we have successfully deployed and optimized our ML algorithms, meticulously reducing the risks associated with false flagging network activity and ensuring real-time inference. The Cato algorithms monitor network traffic in real-time while maintaining low false positive rates and high detection rates.
How Cato Uses Deep Learning to Enhance Threat Detection and Prevention
Using DL techniques, Cato harnesses the power of artificial intelligence to amplify the effectiveness of threat detection and prevention, thereby fortifying network security and safeguarding users against diverse and evolving cyber risks. DL is used in many different ways in Cato SASE Cloud.
For example, we use DL for DNS protection by integrating deep learning models within Cato IPS to detect Command and Control (C2) communication originating from Domain Generation Algorithm (DGA) domains, the essence of our launch today, and DNS tunneling. By running these models inline on enormous amounts of network traffic, Cato Networks can effectively identify and mitigate threats associated with malicious communication channels, preventing real-time unauthorized access and data breaches in milliseconds.
Eliminate Threat Intelligence False Positives with SASE | Download the eBookWe stop phishing attempts through text and image analysis by detecting flows to known brands with low reputations and newly registered websites associated with phishing attempts. By training models on vast datasets of brand information and visual content, Cato Networks can swiftly identify potential phishing sites, protecting users from falling victim to fraudulent schemes that exploit their trust in reputable brands.
We also prioritize incidents for enhanced security with machine learning. Cato identifies attack patterns using aggregations on customer network activity and the classical ML Random Forest algorithm, enabling security analysts to focus on high-priority incidents based on the model score.
The prioritization model considers client group characteristics, time-related metrics, MITRE ATT&CK framework flags, server IP geolocation, and network features. By evaluating these varied factors, the model boosts incident response efficiency, streamlining the process, and ensures clients’ networks’ security and resilience against emerging threats.
Finally, we leverage ML and clustering for enhanced threat prediction. Cato harnesses the power of collective intelligence to predict the risk and type of threat of new incidents. We employ advanced ML techniques, such as clustering and Naive Bayes-like algorithms, on previously handled security incidents. This data-driven approach using forensics-based distance metrics between events enables us to identify similarities among incidents. We can then identify new incidents with similar networking attributes to predict risk and threat accurately.
How Cato Uses AI and ML in Asset Visibility and Risk Assessment
In addition to using ML for threat detection and prevention, we also tap AI and ML for identifying and assessing the risk of assets connecting to Cato. Understanding the operating system and device types is critical to that risk assessment, as it allows organizations to gain insights into the asset landscape and enforce tailored security policies based on each asset’s unique characteristics and vulnerabilities.
Cato assesses the risk of a device by inspecting traffic coming from client device applications and software. This approach operates on all devices connected to the network. By contrast, relying on client-side applications is only effective for known supported devices. By leveraging powerful AI/ML algorithms, Cato continuously monitors device behavior and identifies potential vulnerabilities associated with outdated software versions and risky applications.
For OS Type Detection, Cato’s AI/ML capabilities accurately identify the operating system type of agentless devices connected to the network. This information provides valuable insights into the security posture of individual devices and enables organizations to enforce appropriate security policies tailored to different operating systems, strengthening overall network security.
Cato Will Continue to Expand its ML/AI Usage
Cato will continue looking at ways of tapping ML and AI to simplify security and improve its effectiveness. Keep an eye on this blog as we publish new findings.